SciNet’s publication about Niagara deployment
August 3, 2019 in blog, blog-general, blog-technical, for_press, for_researchers, for_users, frontpage, news, Road_to_Niagara
Have you ever wondered how a supercomputer is designed and brought to life?
Read SciNet’s latest paper on the deployment of Canada’s fastest supercomputer: Niagara.
Niagara is currently the fastest supercomputer accessible to academics in Canada.
In this paper we describe the transition process from our previous systems, the TCS and GPC, the procurement and deployment processes, as well as the unique features that make Niagara a one-of-a-kind machine in Canada.
Please cite this paper when using Niagara to run your computations, simulations or analysis:
“Deploying a Top-100 Supercomputer for Large Parallel Workloads: the Niagara Supercomputer”, Ponce et al, “Proceedings of PEARC’19: Practice and Experience in Advanced Research Computing on Rise of the Machines (Learning)”, 34 (2019).
Learn more about SciNet’s research and publications by visiting the following link.
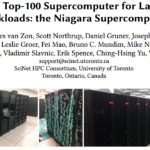
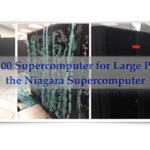